Fewer topics are more top of mind for the user research community than artificial intelligence (AI). And it’s not just us: Merriam-Webster selected “authentic” as its 2023 word of the year in large part because of the consequences of AI—dis/mis information, deep fakes, and the crisis of identity between human and artificial minds.
And yet, 77% of surveyed UX researchers report using AI for some part of their workflow.
User Interviews recently partnered with Maze to bring together two senior leaders thinking about and working on AI in UX research. Jonathan Widawski (Co-founder and CEO at Maze) and Sherwin Yu (VP of Engineering at User Interviews) unpacked their unique perspectives on AI, discussed how their teams are strategizing to use this technology, and outlined how UX researchers can best navigate the hype to make informed decisions for their practice.
Here are 5 key takeaways from that conversation.
1. Artificial intelligence enhances the need for human intelligence
AI’s speed and scale will level the playing field for bringing new products to market. The importance then, as it is now, will be to prioritize market-fit, user needs/jobs, and an interface that is accessible, useful, and intuitive. In short, AI spotlights how important it is to build the right products.
The insights required to build the “right” products derive from—you guessed it—user research.
If AI can reduce build time, increase prototype development, and uncover new feature potentials, the humans—product managers, designers, and certainly researchers—will be a decisive check. As more and more tools flood the market claiming to be “powered by” or “built on” AI, the difference will be UX. And good UX (at least when humans are the end users) still requires human-centered design and research practices.
“We’re calling the new capacity to build ‘time to right.’ We’ve moved from a world where what mattered most was how fast we could push something to market to how fast we can understand our users’ needs, so that we can push the right product to market. With this shift, user insight becomes the only thing that can’t be automated in the process. Because just about anyone can build things fast, user research needs to be the centerpiece of any thriving organization.” ~Jonathan Widawski
This, however, is an argument that must be made and maintained. The pressure will be to “most fast and…” It is our role to complete that sentence with “ensure we meet our users’ needs.”
2. AI will augment researchers’ work, not replace researchers themselves
AI will continue supporting research workflows, creating more space for the human elements inherent to user research, design, and product management. This might be better conceptualized as an always-on intern, speeding up or smoothing repeated tasks.
“I think of AI for my team like an intern, in the sense that they can accomplish many tasks, but you’ll still have to review their work. And honestly, between the time it takes to specify what you want the AI to do and reviewing the work, there are times where it’s a lighter lift to just do the work yourself. Again, it’s still very early days for this technology.” ~Sherwin Yu
These tasks might include:
- Generating top line summaries of customer interviews
- Creating alternative screener or survey questions
- Digesting product documentation to inform study briefs
- Creating alternative formats for research deliverables
- Identifying emerging customer segments to recruit
It will be up to the user researchers and wider human-centered community to iterate, evolve, and revisit where artificial intelligence can reliably, safely, and predictably support processes. In addition, the tools and software providing these models will require careful consideration and vetting by those who leverage them.
Want tips on using AI for qualitative analysis? Give this episode of our Awkward Silences podcast a play.
3. Beware the AI add-ons
Both Sherwin and Jo reiterated how much difficult work goes into adding or infusing research tooling with artificial intelligence. Many of the “AI tools” that have recently cropped up fall short on quality, consistency, or trustworthiness. This is due to a lack of consideration by some platforms. Specifically, they have not asked the crucial question: how will artificial intelligence help our users (see above about the need for more human intervention).
“I am seeing a lot of hammers looking for nails. By that I mean companies slapping “AI” on a feature or an update, but without thinking about how it will impact and affect the user. Any healthy company should be asking about how AI is/will/might change their category and how can I get in front of that change.” ~Jonathan Widawski
“The best practices for building and designing around and for AI are still being defined. Because of that, I think there are benefits of waiting, studying, and watching the early effects of this technology for product builders. So much of the early AI features are chat-based, but chat isn’t the ideal way of interacting for all use cases.” ~Sherwin Yu
Without a bright line between the feature and a real user need, the use of AI in research software will fail to move beyond a gimmick. This means product and engineering managers, as well as their senior leadership, need to consider things like “AI preparedness”—which is the extent to which the current data flows, structures, and formats can adequately leverage AI.
This is to say nothing of processes for maintaining data privacy, customer consent, and tracking of problems. Never forget that it is very, very hard to reverse course after a rapidly-scaling technology like AI is deployed onto and throughout existing infrastructure.
If building products, test and test again. If evaluating a tool with AI features, do some homework on how they work and why they were created in the first place. Talk to customers, collaborate with data and intelligence teams, and always ask, “How does this help our users accomplish what we empower them to do?”
4. Build for humans, not “synthetic” users
The emergence of composite, “synthetic,” or machine-based users continues to garner attention (mostly negative). Fundamentally, the panelists were suspicious of building a product, shaping a roadmap, and tailoring an experience for folks who are not actual (or would be) users, let alone actual people.
Sherwin allowed that such AI-generated user personas might help in the very early stages of development—checking our intuition or early signals—but reiterated that engineering, product, and design teams would miss the best part of creating experiences: seeing and hearing the delight of end users (a large function of user research teams). More critically, such products need to train their models on data generated by humans if it’s to be useful at all.
“As a community, we focus on creating empathy for the folks using our products. I think relying on synthetic customer data distracts from that goal. These companies promising real insights from composite users miss the point. Humans are not a monolith—designing with that diversity in mind makes products better for everyone.” ~Jonathan Widawski
5. Education prepares for (more) evolution
The panel closed with predictions. I originally offered a “this time next year…” horizon. Both Sherwin and Jo reminded me that in AI development, that’s a millennium. OpenAI’s GPT-4 was only released in 2022!
The pace of AI development is only outstripped by the sense of urgency to act. Whether it’s from funders, customers, or stakeholders, you are likely to be asked about how AI might/should/could be used. Taking the time to educate yourself on both the fundamentals of neural networks, language processing models, and how basic AI systems work should not only prepare you to evaluate its use to your workflow, but will be required when responding to requests and evaluating developments.
AI will change the work of product development, research, and design. Exactly how and to what extent is still in flux. That flux should bring confidence, not feverishness. Advocating for cross-company education can help raise awareness of AI-related externalities such as biases, exclusion, and privacy concerns.
AI resources for you and your team:
- For developers: Open Data Science has toolkits and projects to help builders of models spot problem sites before they grow to unmanageable sizes.
- For scholarly reading: New York University’s center for responsible AI maintains original research, courses, and tools for folks looking to dig deeper into ethical AI info.
- For the UX of AI: GitLab maintains a handbook of best practices and approaches to conducting user research on AI systems themselves.
- For research on UX and AI: User Interviews surveyed over 1000 UXRs about their uses of and concerns with AI. Check it out for a pulse of AI in our discipline.
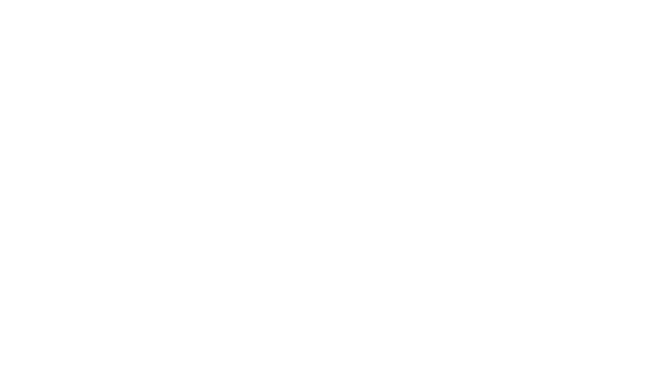