I’ve loved the subject of statistics since my senior year of high school, when I took AP Stats and read Michael Lewis’ revolutionary book about the influence of data in sports, Moneyball. These pushed me towards a career in data science. At my current role at User Interviews, I use data like usage patterns to help tell the story of our company and ensure that we focus on the right things.
The limitations of analytics
I know firsthand how valuable analytics can be for a company; I’ve literally made a living from it. But I also know of its limitations; there are times when you have to dig deeper than possible with a query or a regression model. And often the best way to do this is to simply talk to the people you want to use your product. A truly data-driven organization will complement their analytics with user research, and use learnings from one to power the other.
It’s important to note that there are occasions where it really doesn’t make sense to analyze data using some of the most common statistical methods. First, you might have just launched your product, or perhaps only have a prototype. And even if your product is in an advanced state, you might be adding new features that don’t exist yet. In these cases, it’s likely that you don’t quite have the sample sizes necessary for a rigorous quantitative analysis. Here, user research can help teams figure out if they’re on the right track, and guide them as they build towards the data-collection phase.
Of course, qualitative research doesn’t stop being valuable as soon as you have sufficient data to run a t-test. It’s enlightening to watch a user researcher interview a user and realize just how differently they use the product than we do. At Facebook, where I worked prior to User Interviews, some of the best product ideas came from observing the workarounds users created when the product lacked some functionality. For example, researchers learned that many people would screenshot posts in their feed so they could return to them at a more convenient time, spurring Facebook to build more tools for saving content and finding it again later. These insights might never have emerged if the company hadn’t been talking to its users.
How UXR and Analytics can support each other
UX research can also support analytics teams, and vice versa. A/B testing is a common method to run experiments and determine how different versions of your product perform on certain metrics. At Microsoft, we had a rule of thumb that about a third of your experiments will go as you’d expect, a third will go the opposite way, and the remaining will be inconclusive and require further tests. Additional user research is often necessary for the latter two scenarios to understand why the data moved the way it did. But it’s also important even in the scenario where your gut was correct, because you want to make sure there aren’t further improvements you can make that you might not have thought of.
For example, at Facebook, I worked on a product called the “Local Bookmark,” where we’d suggest new events and restaurants to people.

We’d test different recommendation algorithms, like “Trending Restaurants” or “Places Your Friends Like.” The results would often surprise us, and our user researcher would dig in a bit more. For example, we learned that people don’t automatically trust their friends’ tastes—rather, they trust experts, or a specific set of friends whom they believe make the best suggestions.
Data science lends strength to user research
Data scientists should also support the user researchers on their team. One of the most important parts of product analytics is looking into trends that might not yet be on anybody’s radar. What actions do your most frequent visitors take? Do new users use one part of the site or app more frequently? What features are being used more over time? And, after discovering some of these trends, the analyst should pass the learnings on to a researcher, so that they can actually talk to some of those users and better understand why these behaviors are emerging.
Right after I started at User Interviews, I ran a regression analysis to better understand how researchers decide whether to continue using our products or not. As part of this project, I produced a list of companies that had used us frequently before abruptly stopping; a teammate and I conducted interviews with a few of them to better understand why. As it turned out, the reason didn’t have much to do with User Interviews directly— many of these researchers had simply switched jobs, and nobody at their previous company had picked up the baton. This led us to brainstorm ways to better support more people in a company beyond just the person who initially signed up. For instance, we launched a reminder email for new folks to create an account when a colleague invites them to join a project:

Today, the biggest companies in the world use a blend of user research and data science to learn everything they can about their customers and their market. It’s not an either/or proposition; rather, both disciplines are essential and can continuously strengthen each other. As a data scientist, I understand the value of unlocking insights about thousands and thousands of customers at once. But it cannot replace the surprising results that emerge when you talk to those customers one-on-one.
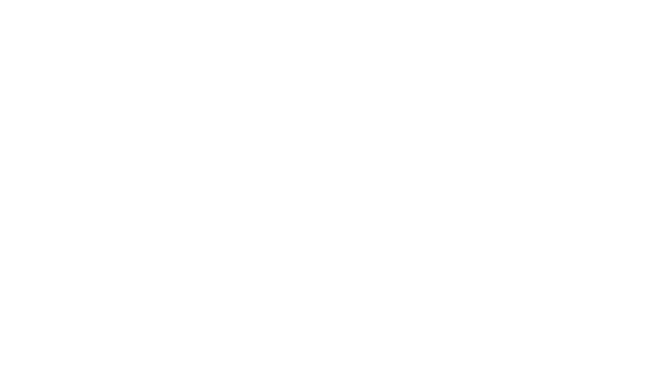